The Ultimate Guide to Data Labeling Platforms
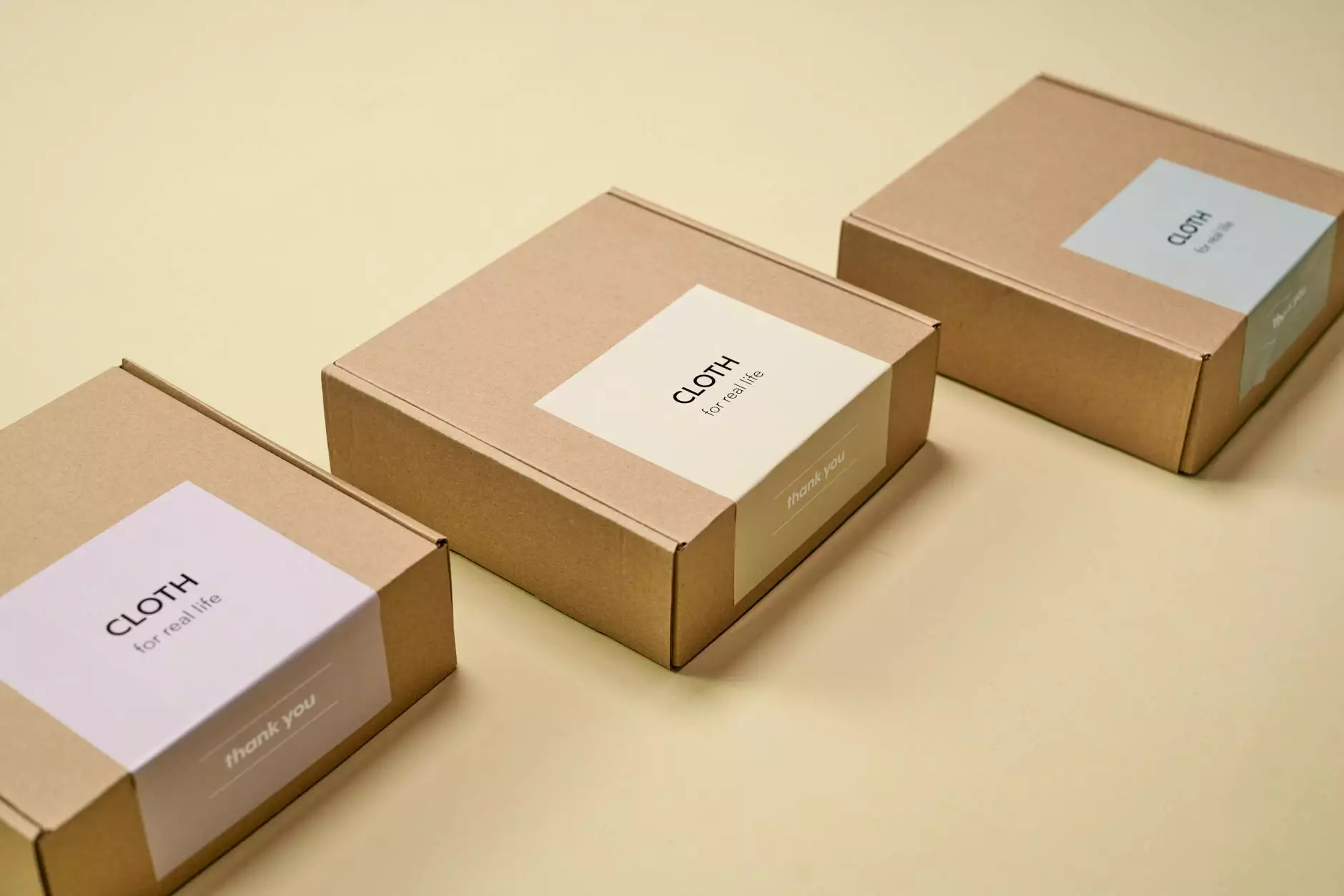
Understanding Data Labeling Platforms
The data labeling platform serves as a crucial backbone for machine learning and artificial intelligence applications. These platforms empower companies by facilitating the annotation of vast datasets that are necessary for training algorithms. Without proper labeling, AI models may struggle with accuracy, leading to inefficiencies and errors in real-world applications.
Why Data Labeling is Important
Data labeling is not just a minor step in the AI model development process; rather, it is an essential component that determines the model’s performance and effectiveness. The quality of data labeling directly impacts the outcomes produced by AI algorithms. Here’s why data labeling is so important:
- Improved Accuracy: Accurately labeled data leads to higher-performing models.
- Enhanced Training: Well-annotated datasets provide clearer guidance for machine learning algorithms.
- Real-world Application: Properly labeled data ensures that AI solutions work effectively in practical scenarios.
Core Features of a Data Labeling Platform
Choosing the right data labeling platform can make a significant difference in the efficiency and effectiveness of your data annotation processes. Here are some core features to consider:
1. User-Friendly Interface
A platform with a well-designed and intuitive interface allows teams to annotate data quickly and accurately, minimizing the learning curve involved.
2. Scalability
The ability to scale your labeling efforts is crucial, especially as your data grows. Look for a platform that can accommodate an increasing volume of tasks without compromising performance.
3. Automation Capabilities
Many modern platforms offer automation features such as pre-annotation, which can significantly speed up the labeling process.
4. Integration with Machine Learning Tools
To ensure a seamless workflow, your data labeling platform should integrate easily with existing machine learning and data processing tools.
Types of Data Labeling
Data can be labeled in various formats, and the choice of labeling type depends on the specific application. Here are some common types of data labeling:
- Image Annotation: Involves identifying and labeling objects within images, which is essential for computer vision applications.
- Text Annotation: Useful for natural language processing tasks, where specific words or phrases are tagged for analysis.
- Video Annotation: Involves labeling frames within video data, commonly used in surveillance and autonomous vehicle training.
- Audio Annotation: Pertains to tagging sounds or speech in audio clips, essential for voice recognition implementations.
Choosing the Right Data Annotation Tool
When looking for a data annotation tool, consider the following factors:
1. Cost-Effectiveness
Assess your budget and the cost of the tools. Many platforms offer flexible pricing models, including pay-per-use and subscription options.
2. Quality of Labeling
Ensure that the platform has a reputation for high-quality labeling services. User reviews and case studies can provide insights into the platform’s effectiveness.
3. Support and Community
Having robust customer support and an active community can facilitate solving issues quickly and learning best practices.
Best Practices for Data Labeling
To achieve the best outcomes from your data labeling efforts, it’s essential to follow established best practices:
- Define Clear Guidelines: Ensure that all annotators understand the labeling criteria and standards.
- Recruit Skilled Annotators: Hiring experienced annotators can greatly enhance the quality of your data.
- Implement Quality Assurance: Regularly check the consistency and accuracy of labeled data to ensure high standards.
- Utilize Feedback: Encourage annotators to provide feedback on the labeling process, which can lead to continuous improvements.
Case Studies: Effective Data Labeling in Action
Case Study 1: Autonomous Vehicles
Companies in the autonomous vehicle industry rely heavily on data labeling platforms to annotate vast amounts of driving data. For instance, by using sophisticated image labeling tools, teams can accurately mark objects such as pedestrians, traffic signs, and lane boundaries. This precise labeling not only enhances the safety of autonomous driving systems but also accelerates their development timeline.
Case Study 2: Healthcare
In the healthcare sector, data labeling plays a crucial role in clinical data analysis. Machine learning models trained with accurately labeled medical images can assist in diagnosing diseases like cancer at early stages. For example, hospitals that have implemented effective data labeling protocols have reported increased accuracy in diagnostic imaging algorithms, significantly improving patient outcomes.
Future Trends in Data Labeling Platforms
The field of data labeling is continuously evolving, driven by advancements in technology and the increasing volume of data generated. Here are some future trends to watch:
1. AI-Assisted Annotation
As AI technologies progress, many data labeling platforms are beginning to integrate machine learning capabilities to assist in the annotation process. This can lead to faster labeling times and reduced human error.
2. Crowdsourced Labeling
Leveraging crowdsourced labor is becoming popular for speeding up the data labeling process. Online platforms that connect businesses with freelance annotators can greatly reduce costs and improve turnaround times.
3. Enhanced Collaboration Features
Future platforms are likely to incorporate enhanced collaboration features, enabling teams to work together seamlessly, regardless of geographical location.
Keylabs.ai: Your Premier Choice for Data Labeling Solutions
At Keylabs.ai, our commitment is to provide a cutting-edge data labeling platform that meets the diverse needs of our clients. We pride ourselves on delivering high-quality, accurate annotations through a combination of advanced technology and skilled human annotators. By choosing Keylabs.ai, you are ensuring that your data is labeled accurately and efficiently, leading your machine learning projects to success.
Our Unique Offerings
Keylabs.ai stands out by offering a range of unique services, including:
- Tailored Annotation Services: Custom solutions designed to fit your specific project needs.
- Performance Analytics: Advanced tools to track labeling progress and quality over time.
- Flexible Integration: Easily integrate with your existing workflows and data processing pipelines.
Conclusion
In the fast-paced world of AI and machine learning, the importance of a robust data labeling platform cannot be overstated. The quality of labeled data directly influences the performance of machine learning models, making it essential for businesses to invest in effective annotation solutions. By adopting best practices and leveraging the right tools, organizations can enhance their data labeling process, ensuring that their AI initiatives lead to unprecedented success.