The Importance of an **Image Classification Labeling Tool** in Today's Business Landscape
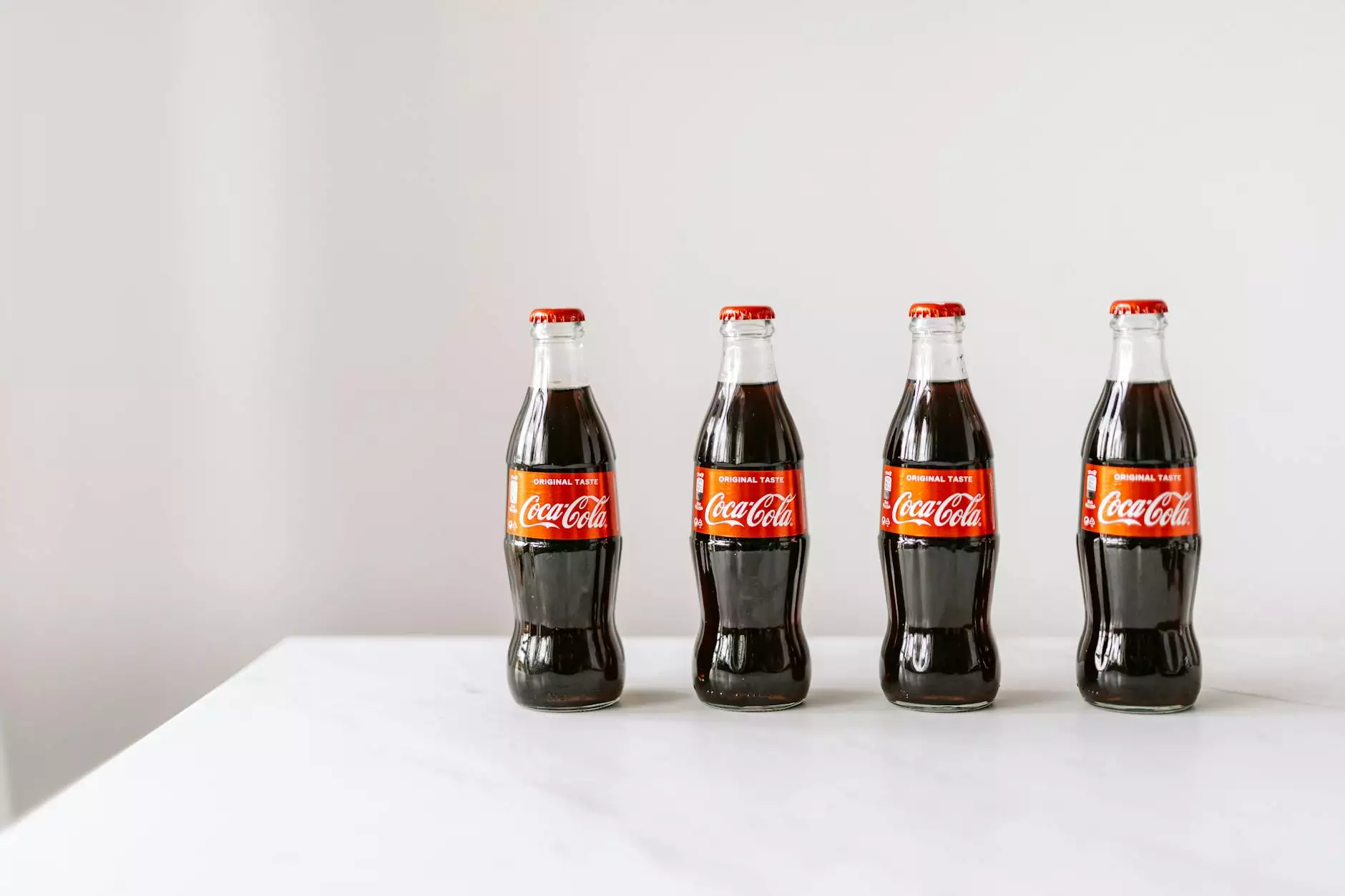
In the rapidly evolving digital age, where data reigns supreme, the ability to effectively manage and classify images is more crucial than ever. An image classification labeling tool serves as an integral part of data processing, especially in industries reliant on accurate visual data. As businesses seek to leverage machine learning and artificial intelligence, understanding the functionality and advantages of such tools becomes paramount. This article explores the necessity, benefits, and implementation of image classification labeling tools in a business environment.
1. What is an Image Classification Labeling Tool?
An image classification labeling tool is software designed to assist in the process of annotating images for the purpose of training machine learning models. These tools enable users to tag and categorize images effectively, ensuring that data is organized and retrievable. The labeling process is essential for supervised learning in artificial intelligence, where algorithms learn from labeled datasets to make predictions or classifications.
Key Functions of an Image Classification Labeling Tool
- Image Annotation: The tool allows users to draw bounding boxes, polygons, and other shapes to highlight areas of interest in images.
- Object Detection: It can identify and classify multiple objects within an image, making it invaluable for industries like retail and automotive.
- Category Mapping: Users can assign custom labels, facilitating the training of neural networks with specific data for various applications.
- Quality Control: Many tools offer features for reviewing and validating annotations to maintain high data quality.
- Integration Capabilities: Most sophisticated tools integrate seamlessly with machine learning frameworks, allowing for a streamlined workflow.
2. The Role of Data Annotation in Business
As businesses increasingly rely on data annotation tools, the integration of effective labeling processes becomes critical. Quality data leads to better-trained models, reducing errors in predictions and classifications. This section delves into why data annotation is a cornerstone of effective business operations.
Benefits of Data Annotation Tools in Business
- Improved Model Accuracy: An accuracy-centric approach in training AI models hinges on the quality of the labeled dataset. Higher quality annotations lead to superior model performance.
- Time Efficiency: Automated annotation processes expedite data preparation, allowing businesses to allocate resources to other important tasks.
- Scalability: Businesses can handle larger datasets effortlessly, adapting quickly to changing market conditions and data needs.
- Cost-Effectiveness: Reducing the time spent on repetitive labeling tasks significantly lowers operational costs.
- Enhanced Collaboration: Many annotation tools offer cloud capabilities, fostering teamwork across different geographical locations.
3. Choosing the Right Image Classification Labeling Tool
With a plethora of options available in the market, selecting the right image classification labeling tool for your organization can be daunting. Various factors must be assessed to ensure that the chosen tool meets the specific needs of your business.
Key Considerations for Selection
- Usability: The interface should be user-friendly, allowing teams to access and utilize the tool with minimal training.
- Customization: Check if the tool allows customization of labeling formats and categories to suit your unique needs.
- Support and Updates: Opt for tools that provide ongoing support and regular updates to keep pace with technology advancements.
- Pricing Model: Evaluate whether the pricing structure is subscription-based, per annotation, or a one-time fee to predict long-term costs.
- Integration: Assess how well the tool integrates with your existing technology stack, especially machine learning frameworks like TensorFlow or PyTorch.
4. Real-World Applications of Image Classification Labeling Tools
The applications of image classification labeling tools extend across various industries. Below are some prominent sectors that have successfully utilized these tools:
Healthcare
In healthcare, image classification labeling tools assist in analyzing medical images such as X-rays, MRIs, and CT scans. Accurate diagnosis through AI-driven models relies heavily on well-annotated datasets, leading to faster disease detection and improved patient outcomes.
Retail
Retailers harness these tools for visual search capabilities, enhancing customer experience by providing image-based product recommendations. By accurately classifying product images, retailers can increase conversion rates and customer satisfaction.
Autonomous Vehicles
The automotive industry employs image classification labeling tools to train AI systems in recognizing pedestrians, road signs, and other vehicles. This critical functionality aids in developing safer autonomous driving technologies.
Security and Surveillance
In security, these tools help in the identification and classification of anomalies in surveillance footage, enhancing security measures through advanced threat detection and response strategies.
Manufacturing
In manufacturing, image labeling tools are deployed for quality control processes, ensuring products meet specified standards by validating visual aspects through AI models.
5. Workflow of Using an Image Classification Labeling Tool
Understanding the workflow of an image classification labeling tool can illuminate how to implement it effectively within your business operations. Here’s a step-by-step guide:
- Data Collection: Gather the raw image data that needs to be classified. This dataset forms the base of your model's training.
- Annotation Setup: Configure your labeling tool according to the project requirements. Define categories, labeling styles, and guidelines.
- Labeling Process: Use the tool to annotate images. Ensure adherence to quality guidelines for maximum data efficiency.
- Quality Assurance: Regularly review labeled data to rectify errors and ensure accuracy and consistency within the dataset.
- Exporting Data: Once labeling is complete, export the dataset in a format compatible with your machine learning framework.
- Model Training: Utilize the labeled dataset to train your machine learning models, adjusting parameters as necessary to improve performance.
6. The Future of Image Classification Labeling Tools
The future of image classification labeling tools appears promising, with numerous advancements on the horizon including:
Integration of AI/ML in Annotation
The incorporation of artificial intelligence in annotation tools will facilitate automatic labeling, significantly reducing the manual workload involved in data preparation.
Increased Usability
User experience will continue to evolve, with intuitive interfaces leading to greater accessibility for non-technical professionals and teams.
Collaborative Platforms
Future tools may offer enhanced collaborative features, allowing teams from different locations to work on projects seamlessly and in real time.
Enhanced Customization
As industries become more specialized, tools will need to offer greater customization options to meet the unique needs of various sectors.
Conclusion
The significance of utilizing an image classification labeling tool cannot be overstated in today’s data-centric world. Companies must invest in high-quality labeling tools to obtain accurate, reliable datasets that empower their AI and machine learning applications. This investment not only leads to improved operational efficiency but also enhances decision-making processes across various sectors. Businesses that harness the capabilities of these tools, such as those offered by keylabs.ai under categories like *Data Annotation Tool* and *Data Annotation Platform*, are better positioned to thrive in a competitive landscape where data dictates success.
By understanding the functions, benefits, and best practices associated with image classification labeling tools, organizations can take significant strides towards optimizing their data workflows and leveraging artificial intelligence effectively.